UW-Madison cloud computing research moves into new phase
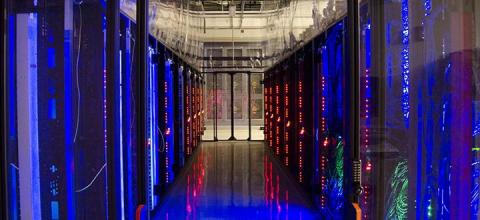
The vast computing structure, or cluster, maintained by the three campuses in CloudLab serves as a “testbed” or means for others to run experiments on. Submitted image
The University of Wisconsin–Madison is part of a team of campuses receiving nearly $10 million, collectively, from the National Science Foundation (NSF) to further develop cloud computing infrastructure and enable high-level research by scientists around the country.
The grant represents phase two of an effort called CloudLab, a partnership with the University of Utah and Clemson University in South Carolina. In 2014, NSF awarded $10 million to support CloudLab’s first phase.
Cloud computing allows users of technology to make use of remote, shared infrastructure and services. Its impact on our daily lives, as well as complex scientific research, has grown exponentially in recent years.
UW-Madison computer science Professor Aditya Akella serves as principal investigator on the Wisconsin end of the project. The University of Utah is the lead campus.
As Akella explains, the vast computing structure, or cluster, maintained by the three campuses serves as a “testbed” or means for others to run experiments on. “We want people to use the cluster in unexpected ways and make discoveries. CloudLab has met that charter in the past, and we hope to make it even better in the future,” he says.
One measure of CloudLab’s success to date is its heavy use by researchers. More than 56,000 experiments are currently running on the testbed, and Wisconsin’s servers are frequently operating at 90 percent of capacity.
CloudLab’s new phase will provide state-of-the-art support for machine learning, a branch of computer science in which computers “learn” from data without being explicitly programmed to do so. CloudLab will employ computer chips called GPUs (Graphics Processing Units) that are well suited to cutting-edge machine-learning research.
“A lot of machine learning advances in recent years in image classification, machine translation and robotics have happened because of training at large scales on clusters of GPU-equipped machines. The research community sees value in using these kinds of computer clusters,” notes Akella.
The new phase also includes state-of-the-art “whitebox” network switches that offer experimenters the ability to reprogram, on the fly, how their network supports applications.
The new round of funding began Oct. 1 and will span a three-year period.
Tags: computer science, grants, research