Machine learning can detect a genetic disorder from speech recordings
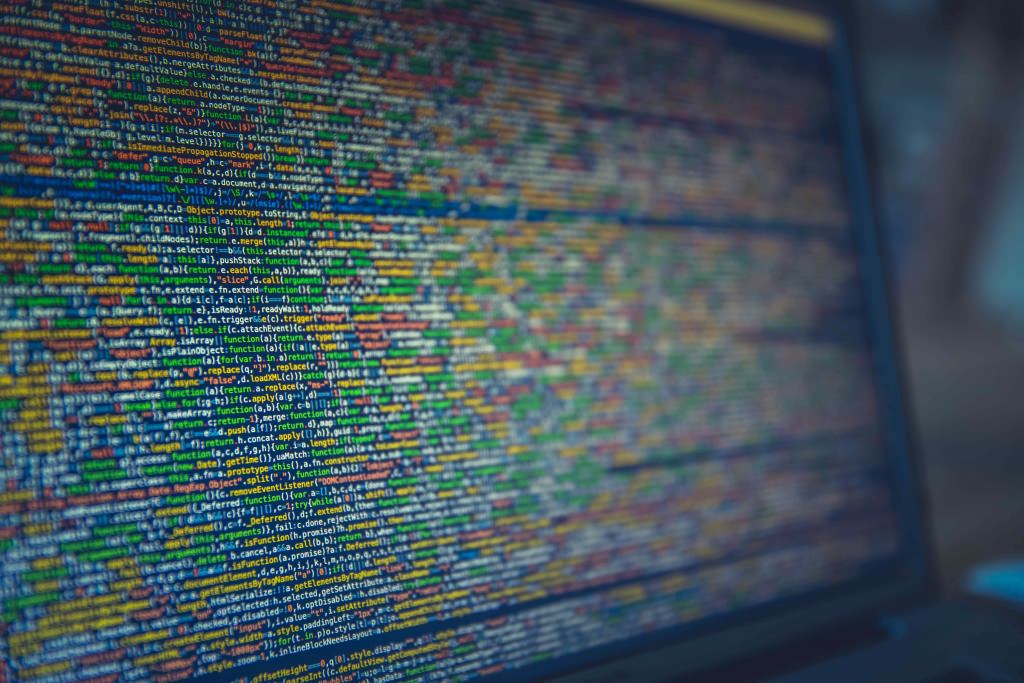
Machine learning is a form of artificial intelligence by which algorithms are “trained” to analyze new information using existing data. Researchers are using it to identify individuals with a genetic condition known as fragile X premutation. Creative Commons
How much information can we extract from a five-minute recording of someone talking? Enough to tell whether that individual may be genetically predisposed to some health complications, according to researchers at the University of Wisconsin–Madison’s Waisman Center and Wisconsin Institute for Discovery.
In a new study published this month in Scientific Reports, the researchers used machine learning to analyze hundreds of voice recordings and accurately identify individuals with a genetic condition known as fragile X premutation, which increases the risk of developing neurodegenerative disorders, infertility or having a child with fragile X syndrome.
While fragile X syndrome — characterized by intellectual disability and behavioral, physical and learning challenges — is relatively rare, millions of people across the world have fragile X premutations. “But the premutations remain underdiagnosed, and people are often unaware of their increased health risks,” says Marsha Mailick, professor of social work and UW–Madison vice chancellor for research and graduate education. Mailick is a co-author of the study.
Part of the challenge in diagnosis is that the genetic testing to identify fragile X premutations can be time-consuming and resource-intensive. “Our group of researchers wanted to develop a method to quickly and cost-effectively screen for this condition,” says Mailick.
That led them to machine learning — artificial intelligence computer programs that can be “trained” using existing data sets and then used to analyze new information.
“We can go from taking hours to analyze and annotate each recording to needing less than a second,” says Kris Saha, assistant professor of biomedical engineering at UW–Madison and the study’s senior author.
The researchers focused on voice recording analysis because Mailick and her colleagues have shown in prior studies that this can yield valuable information about the families of individuals with fragile X premutations.
For instance, in 2012, a study led by the UW’s Jan Greenberg, professor of social work and associate vice chancellor for research and graduate education, analyzed five-minute recordings of mothers talking about their children with fragile X syndrome. The study showed that parental warmth and a positive family atmosphere were associated with fewer behavioral problems in their children. Greenberg is a co-author of the new study.
Another co-author, Audra Sterling, an assistant professor of communication sciences and disorders at UW–Madison, used the same recordings to show a strong correlation between age and specific speech difficulties in middle-aged and older women with fragile X premutations. These findings indicated that voice recordings could be used to track the development of cognitive challenges faced by many older individuals with fragile X premutations.
But according to Mailick, coding the speech characteristics was time consuming and required clinical expertise, neither of which are needed with the method reported in the new study.
“We have a rich legacy of research on fragile X syndrome at UW–Madison,” says Mailick, “and only in interdisciplinary environments, such as at the Waisman Center, where all co-authors are long-term affiliates, could the needed expertise and data come together for this research.”
Saha, Greenberg, Sterling, Mailick, and graduate student Arezoo Movaghar, joined forces to design and implement initial machine learning algorithms that could distinguish between two groups: mothers with fragile X premutations and those without.
The researchers used 100 five-minute recordings of mothers with fragile X premutations talking about their children with fragile X syndrome, and as a comparison data set, another 100 recordings from mothers of children with autism spectrum disorder.
These two groups were chosen because families with children with disabilities often face distinct challenges and stresses compared to families with typically developing children, says Movaghar, who is the first author of the study.
Based on transcripts of the recordings, and using machine learning algorithms, the researchers created a list of language and cognitive features, such as the average length of sentences in the recording or the number of filled pauses — vocalizations, such as “um,” “ah,” or “oh.” They found some of these features more useful in distinguishing between the two groups.
In fact, using the most informative features, the machine learning algorithms could distinguish between mothers with fragile X premutations and mothers without the premutation with 81 percent accuracy.
According to calculations by the researchers, machine learning-based screening followed by confirmatory genetic tests would save more than $11 million compared to using genetic tests alone to identify 1,000 women with fragile X premutations in the general population.
This work is a first step toward a quicker, more cost-effective screening process, says Mailick. “We plan to expand into screening other populations, such as men with fragile X premutations.”
And the machine learning algorithms developed in this study don’t have to be limited to health conditions associated with fragile X premutations. “What’s also exciting is the possibility of using similar algorithms for other disorders,” says Saha.
Moving forward, “we want to streamline the way we collect the data,” says Movaghar, who is working to develop a mobile app to accomplish this goal. “It would ask a series of simple personal and medical questions, and then record a five-minute voice sample,” she says. Data could even be sourced from ubiquitous audio recordings on smartphones or smart speakers in the home.
Then the machine learning algorithms would get to work.
This project was supported by National Institute of Aging (Grant number R01 AG08768), National Institute of Child Health and Human Development (Grant numbers R01 HD082110, and P30 HD03110-40-S1), and the National Institute on Deafness and other Communication Disorders (Grant number R03 DC011616). We are also grateful for the support received from Wisconsin Alumni Research Foundation (WARF) and Waisman Center under Grant number U54 HD090256 and P30 HD03352 and the Graduate School at UW–Madison (Interdisciplinary Grant).