Machine learning and its radical application to severe weather prediction
In the last decade, artificial intelligence (“AI”) applications have exploded across various research sectors, including computer vision, communications and medicine. Now, the rapidly developing technology is making its mark in weather prediction.
The fields of atmospheric science and satellite meteorology are ideally suited for the task, offering a rich training ground capable of feeding an AI system’s endless appetite for data. Anthony Wimmers is a scientist with the University of Wisconsin–Madison Cooperative Institute for Meteorological Satellite Studies (CIMSS) who has been working with AI systems for the last three years. His latest research investigates how an AI model can help improve short-term forecasting (or “nowcasting”) of hurricanes.
Known as DeepMicroNet, the model uses deep learning, a type of neural network arranged in “deep” interacting layers that finds patterns within a dataset. Wimmers explores how an AI system like DeepMicroNet can supplement and support conventional weather prediction systems.
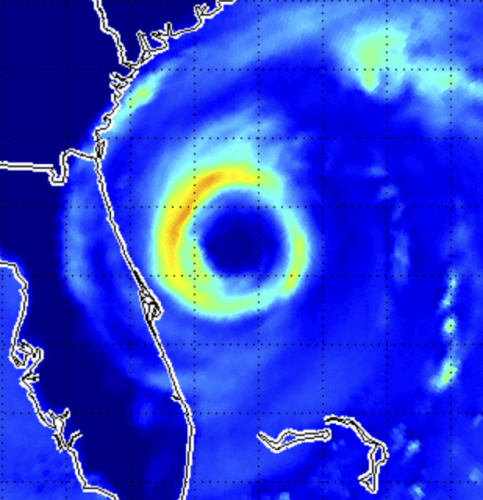
A microwave image of Hurricane Dorian. According to UW–Madison Cooperative Institute for Meteorological Satellite Studies scientist Anthony Wimmers, machine learning could enable forecasters to make better predictions about the intensity of tropical systems like Hurricane Dorian using microwave satellite images like this one. UW–Madison CIMSS
In a 2019 paper published in the journal Monthly Weather Review, Wimmers and colleagues Chris Velden, of CIMSS, and Josh Cossuth, of the U.S. Naval Research Lab, describe a way to harness deep learning to estimate hurricane strength based on data from particular weather satellites. As they pass overhead, these satellites collect key information about a hurricane’s structure through measurements in the microwave part of the electromagnetic spectrum. With 30 years of microwave data, the paper demonstrates how the model can estimate — with increasing accuracy — a tropical cyclone’s intensity.
“The results from the study show a lot of promise, not just for the model’s nowcasting accuracy, but also because these results came from data not typically used to estimate hurricane intensity,” Wimmers says.
Rapid analyses like these can give forecasters vital information about a storm’s behavior and what to expect, such as whether the storm will undergo eyewall replacements or rapid intensification.
When predicting a hurricane’s maximum sustained winds, DeepMicroNet’s results differed from the historical record of forecaster-estimated values by about 16 miles per hour. DeepMicroNet’s results improved, however, when the datasets were limited to data measured directly by aircraft. Then, DeepMicroNet was off by less than 11.5 mph. By comparison, estimates using state-of-the-art methods are typically off by around 10 miles per hour.
Rapid analyses like these can give forecasters vital information about a storm’s behavior and what to expect.
Wimmers set out to answer three main questions. First, he wanted to determine how well the model performed compared to state-of-the-art methods for predicting hurricane intensity. Second, it was important to assess whether the results were meaningful and advanced the science of meteorology. Lastly, he wanted to demonstrate new ways to incorporate less commonly used data such as microwave imagery into prediction models, while offering valuable insight into a storm.
“The reason deep learning systems have grown so much in satellite meteorology is because they are ready-made for these types of applications, where you have tens of thousands of images available for training a model,” says Wimmers. “It also applies to situations where you need an answer rapidly.”
Wimmers designed his experiment to test how well an AI system could reproduce a hurricane’s history. The historic hurricane intensities came from a combination of forecaster estimates based on other satellite data and aircraft observations. With no knowledge of the data types, DeepMicroNet’s job was to estimate intensities from a large, independent dataset of microwave imagery measured in the 37 GHz and 89 GHz frequencies.
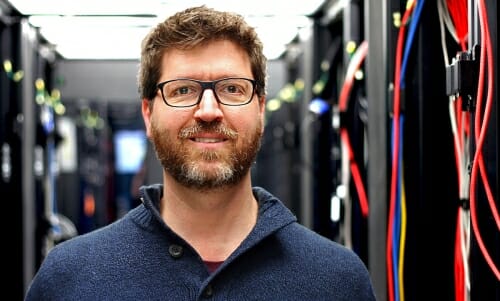
Anthony Wimmers’ latest research investigates how an AI model can help improve short-term forecasting of hurricanes. Photo: Eric Verbeten
“Those two frequencies are useful for revealing different hurricane structures,” says Wimmers. “Their relatively coarse resolution also means they can be analyzed and processed in a computer quickly.”
Wimmers’ AI program is capable of churning through more than 50,000 hurricane images in under two hours. It was coded using Python, a programming language that has become the standard for powerful machine learning applications. Wimmers says these systems reach peak performance after running at least tens of thousands of examples. Through repetitive processing of training images, the system showed it could detect and memorize patterns in a hurricane’s structure.
DeepMicroNet delivered the final results after a validation test using a smaller subset of only 3,000 images. Here it applied what it learned during training and accurately assessed the intensity of the tropical cyclones. In the past, running models with large datasets could take as long as a week to compute. Today, though, advances in computing have reduced a training task like DeepMicroNet’s to 90 minutes.
“These results were a promising demonstration of the kinds of things we can do with machine learning in the future,” says Wimmers. “We can interpret the results of deep learning networks to improve our physical models. We can find patterns that used to be beyond our reach because they were too complicated.”
The field of AI research is evolving so rapidly that Wimmers says it can be a challenge to stay current. However, his work is on the leading edge of using artificial intelligence as a tool for improving weather forecasts.
While deep learning systems can have powerful predictive capabilities, their design has an inherent drawback. Outside the AI community, the words “black box” are often used to describe AI systems and their results. A source of lengthy debate, “black box” refers to how difficult it can be, at times, to retrace the path an AI model took to reach its conclusion. It presents a major problem for the scientific community — one that is built on transparency and reproducibility.
Wimmers argues that despite some of AI’s opaque methodologies, researchers have much to gain by probing AI systems and their processes.
“On one hand, an image processing deep learning model can tell you quite a bit about itself based on its performance, or where it focused its efforts and which areas of an image were of highest consequence,” says Wimmers. “But on the other, we don’t have a good system for translating all that information into its basic physics and interpreting that to tell us what is happening in the natural world.”
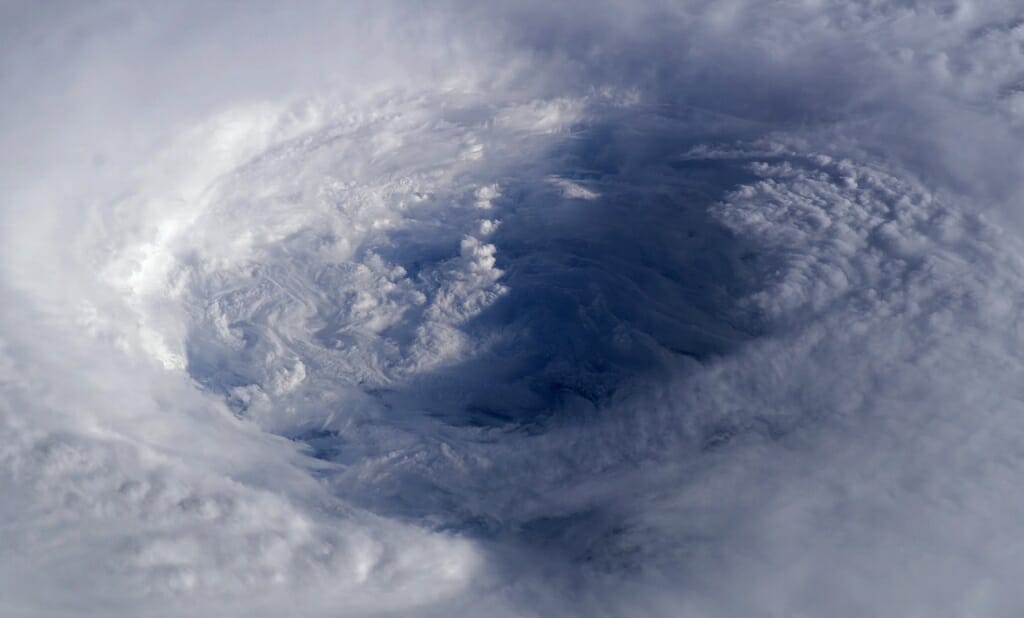
The eye of Hurricane Isabel. Researchers at the UW–Madison Cooperative Institute for Meteorological Satellite Studies and the U.S. Naval Research Lab are exploring ways in which machine learning could help improve weather forecasting for severe weather, such as hurricanes. NASA
More traditional weather models are based on a series of equations and datasets derived from the physics of the atmosphere. By contrast, an AI system often ignores any existing assumptions and focuses solely on finding patterns in the data. In the best-case scenario, the AI system incorporates natural processes in the atmosphere that were previously overlooked by traditional models.
The AI findings, then, could be used to supplement current weather models and reveal trends worth further investigation. Wimmers sees both approaches playing complementary roles, each with their strengths and weaknesses.
“While (conventional weather models) and deep learning models already share a lot of similarities in how they function, they are two different tools that serve different purposes, and we can make use of both,” he says.
The field of AI research is evolving so rapidly that Wimmers says it can be a challenge to stay current. However, his work is on the leading edge of using AI as a tool for improving weather forecasts. CIMSS Director Tristan L’Ecuyer sees AI playing an increasingly important role for a number of areas in the atmospheric sciences, such as identifying severe weather, identifying turbulence, predicting lake-effect snow, measuring air motion, and tracking sea ice movements.
“Now that high-resolution satellite images are captured every few minutes and generating enormous volumes of data to analyze, there is a need to develop innovative new ways of extracting practical information from them,” says L’Ecuyer. “AI is going to play a critical role in the transition from data gathering to information production and action in the next decade and CIMSS is positioning itself to lead this effort in the coming years.”
This work was supported by the U.S. Naval Research Lab.
Tags: computers, meteorology, research, weather